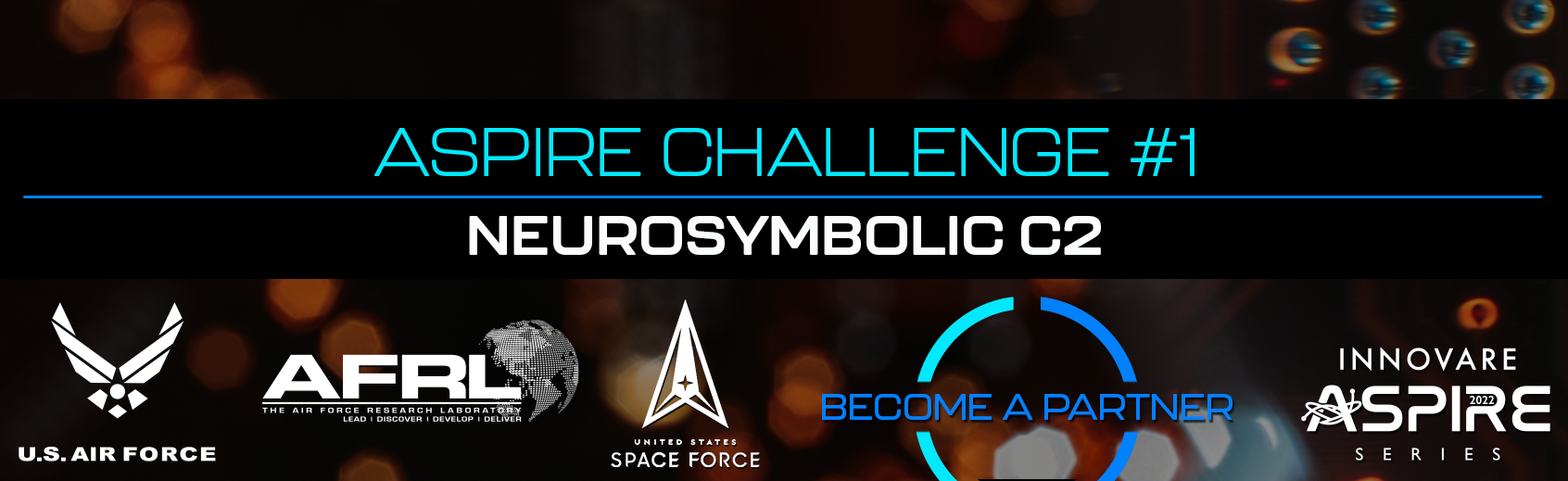
Neurosymbolic C2

What is the objective?
To develop next-generation artificial intelligence (AI) solutions that integrate neural learning systems and formal symbolic reasoning for more efficient autonomous decision making, by the adoption of these State-of-the-Art techniques and applying them to a variety of military command & control missions and broader industrial applications, such as warehouse robotics and autonomous driving systems.
What problem are we trying to solve?
Current simulation environments are too slow for the adoption of state-of-the art machine learning approaches to autonomous decision-making, such as those proposed in the areas of reinforcement learning and planning. Recent noteworthy examples include the defeat of the world Go champion and expert-level performance in multi-agent real-time strategy settings. Despite their success, these approaches require tens of millions of simulation runs or tens of thousands of years’ worth of simulation data. These data requirements preclude the adoption of such data-hungry methods for military and industrial applications. Some of these challenges are being addressed under the umbrella of what is now called the “third wave” of AI, otherwise known as neurosymbolic AI. This entails the integration of neural learning systems and the mathematically principled reasoning of symbolic structures. In this vein,we envision a future for neurosymbolic autonomy wherein the integration of formal symbolic structures and deep reinforcement learning usher in a new era of data-efficient decision-making. Furthermore, this integration of the neural and the symbolic can mitigate challenges of uncertainty reduction, partial observability, and data discrepancies between simulation environments and real-world use cases.
What outcome do we hope to achieve?
To develop novel neurosymbolic reinforcement learning and planning solutions that reduces the data requirements of autonomous decision making applied to complex, large scale, and dynamic environments, such as JointAll-Domain Command and Control (JADC2), and unify the powerful data-driven neural perception of observed data via neural networks and the precise conceptually-driven symbolic reasoning of perceived information.
What resources could the lab provide?
The lab can provide relevant target environments such as the Advanced Framework for Simulation, Integration and Modeling (AFSIM) and the Air Warfare Simulation Model (AWSIM) as well as identify surrogate environments,subject-matter expertise, and evaluation criteria by which to evaluate the effectiveness of the proposed neurosymbolic approach.
What would success look like?
A reduction in the data requirements to deal within an uncertain environment to enable state-of-the-art autonomous decision-making methods by two orders of magnitude while maintaining similar performance. The performance metric is dependent on the environments chosen and can include things like maximizing a reward signal, yielding explainable actions, or establishing robust control policies, among others.
What types of solutions would we expect?
To address potential solutions, it is first worth noting the technical challenges encountered in autonomous decision-making, such as the area of reinforcement learning, when compared to more conventional data analytics problems such as classification and regression. First, the actions enacted by the agent(s) have the capacity to change the data observed in the future via changes in the modelling and simulation (M&S) environment. For example, if an agent decides to shoot down an Unmanned Air Vehicle (UAV), that UAV will not be seen again in the environment in the future. Second, the agent must reason about a long-term objective accomplished as the result of multiple actions. Thus, the types of neurosymbolic autonomous solutions we expect would have to address these challenges. Potential candidates include the co-learning of control policies and symbolic semantics to capture data and goal representations, respectively, though many other potential solutions exist andwe welcome creative and novel approaches. The industry and academic partners may develop their own M&S environment for experimental purposes or leverage an existing simulation environment (e.g., StarCraft 2, OpenAI Gym, RAND’sAFGYM, etc.).
What's in it for industry?
There will be a cooperative research agreement where both the industry or academic team and the government will benefit from the solution. In particular, more efficient autonomous decision making can enable the adoption of powerful learning techniques methods for warehouse robotics and autonomous driving systems by reducing the cost in time and energy budget of training machine learning algorithms. The technologies developed could have large applicability to both civilian and military applications and can be leveraged as part of a future greater submission to an Air Force program orother Department of Defense (DoD) & Government Agencies (e.g., DARPA,Department of Homeland Security).