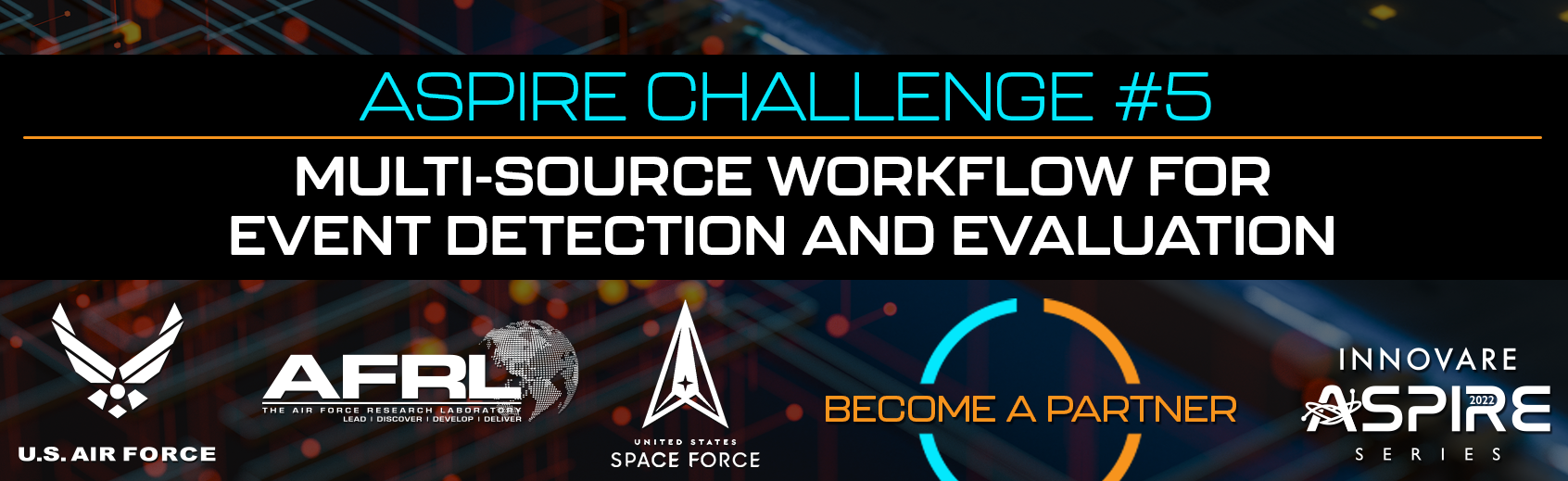
Multi-Source Workflow for Event Detection and Evaluation

What is the objective?
Create a product that can identify and characterize events using the fusion of different types of collection (multi-INT), from different sources (multi-source), and including different representations of data modes (multi-modal), starting with object sensing and identification to hypothesis formulation, followed by intelligent request for information, collection, and analysis. Assume data integrity, the accuracy, completeness, safety and consistency of data is maintained over time and across all formats. The event and dataset itself are open-ended so organizations can develop their own system or workflow.
What problem are we trying to solve?
Decision makers require a problem-solving strategy of breaking down information requirements and intelligence questions into collectibles and observables to develop all-source, multi-INT collection strategies in an automated, machine learning manner. The strategy must ensure the right pieces of data will be in the hands of the right decision maker at the right time. We are taking information (such as sensordata, positional data, etc.), and transforming it, through data fusion, into usable data for analysts to perform situational and threat refinement to then present to decision makers. The figure below, Joint Directors of Laboratories (JDL) Model of Data Fusion is only one example of a model that provides insight of the information we are trying to refine. Upon taking information from sensors, the data is pre-processed to make object assessments, situation assessments, and finally impact assessments. Consider the following scenario: we’re worried about a US space satellite and are seeing indication of adversary movements that are causing us concern. This indication is based on habits in the past, so we call the commander and tell her/him, with a high degree of confidence, that the adversary may launch a ground-based missile against this high value asset. Additional examples include terrorism prediction, law enforcement/emergency response, and environmental changes.
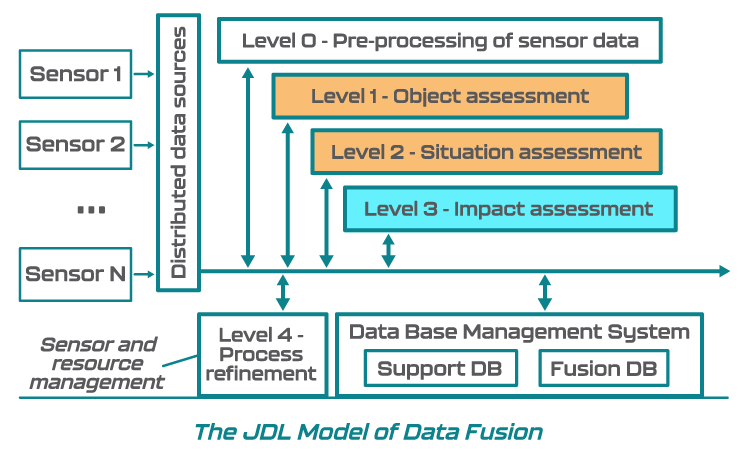
What outcome do we hope to achieve?
Independent of the domain – develop and apply machine learning, machine learned Patterns of Life, normalcy/abnormality models for predictive analytics for single and multiple event assessment and response,identification of key features for multi-modal object models for identification of inconsistent or bad data, identification of time windows for collectibles,and observables necessary to develop information collection strategies and link initiating events with required information. Command and Control (C2) decision-making must be informed, and based on all information present.
What resources could the lab provide?
Single point of contact access to highly educated and experienced professionals with decades-long experience in artificial intelligence, machine learning, and deep learning, as well as strategic insights on how to deliver a relevant product; access to high-power computing and unclassified, publicly available scenarios from DARPA, Defense Against Dark Arts, etc. This project should not be limited to space-based events, rather the product should be applicable to any unclassified dataset (natural disasters,illegal fishing, etc.), and eventually applied to space events.
What would success look like?
End to end software demonstration from data analysis, sensemaking, automated information requests, to actionable information: development and application of artificial intelligence and machine learning (AI/ML) sense-making algorithms for predictive analytics and pattern of life hypothesis generation. Ability to confirm hypothesis to “get left” of events. Application of cutting-edge AI/ML and sense making algorithms. Ability to automatically identify key observables and timelines for intelligent information requests, project potential outcomes, determine threats and potential impact, and improve degree of confidence.
What types of solutions would we expect?
Demonstration of capability through unclassified use cases:identification of collectables and observables necessary for information collection strategies, decomposition of events of interest into observables and indicators, object and information mapping, hypothesis management. Unclassified use case could include: ML algorithms developed for measurement of social reactions in a population, based on social media data. This includes classification and inference of social reactions in specific geographic regions and at varying levels of intensity across the full range of societal behaviors, classification for security concerns such as hostile intent or social unrest, classifying reactions collected from structured and unstructured social media data sources, and ontological classification and inference of social media data.
What's in it for industry?
Aspire Challenge pursuits on this topic are an avenue for industry to solve technical problems on a national and global scale, and can be specifically applied to Department of Defense (DoD), Space Force (USSF), and Air Force (USAF); as well as the opportunity to compete for DoD/USSF/USAF solicitations; access to follow-on collaborative opportunities (e.g., Small Business Innovative Research (SBIR), or Broad Area Announcements (BAAs)). Organizations will also be able to work alongside government, academia, andfellow industry leading partners in the field.